Banks have a longer breath to sit out the generative artificial intelligence innovation phase using their strong balance sheets, which gives them a strategic advantage.
Mantel Group’s ongoing market research and our recently published State of Data & AI 2024 Report reveal four major opportunities and challenges for banking and financial services.
1. Cloud migration will take off in the next 2 years
As regulated entities, banks and financial services institutions have been slower off the blocks in terms of enterprise wide cloud adoption compared to other sectors such as retail, media and entertainment.
That is changing with cloud adoption ramping up as financial services institutions get comfortable with the prudential regulator’s guidance on the use of outsourced computing providers.
The Challenge
This new value chain and outsourced ecosystem requires a fundamentally different approach to data and cyber resilience than the one financial institutions employed when they built all their own technology and housing it on premise in giant server rooms. This old on-premise approach was not necessarily more secure, but it did allow more control.
2. Focus on improved customer experience, efficiency gains and superior decision making
Financial services – banking especially – is increasingly becoming a virtual play. The dramatic reduction in cash transactions, closure of less trafficked physical branches, and the move to online transactions has resulted in perfect alignment in relation to financial institutions technology investments.
Financial institutions suddenly find themselves in a situation where creating amazing customer experiences and increasing operational efficiency both require the same thing: a modern technology stack with advanced data processing and analytics capabilities.
The Challenge
Only 50% of organisations undertaking AI projects indicate they manage to align their AI activities well with business strategy. However, financial services institutions are closing the gap in terms of prioritising high value initiatives. They are assessing AI and machine learning use cases using conventional product management practices to prioritise the biggest prize over quick wins, the loudest voice, or whoever has the ear of the data team.
In addition to breaking down internal silos, this process aligns what customers really need with what data and AI products end up in development.
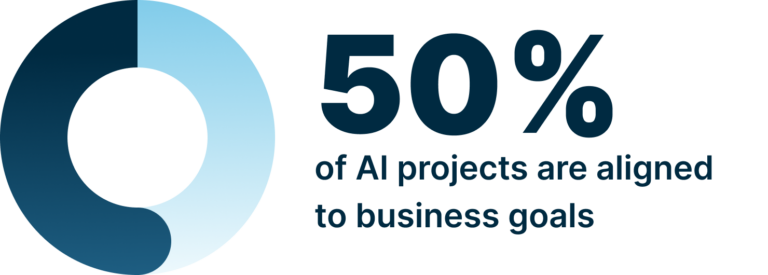
3. Strong appetite to accelerate AI/ML, despite limited adoption so far
Banks and financial services institutions are – and have always been – among the most aggressive adopters of machine learning, and one of the most proactive investors in artificial intelligence, in their pursuit of improving customer experience, driving efficiency gains, and superior risk-based decision making.
Data quality in financial institutions is typically higher than other industries, and stronger data privacy and security standards give these institutions a competitive edge to leverage AI and ML for superior decision making, for instance on credit risk, and asset and liability management. Additionally, they recognise AI is a critical technology in defence against criminals using the same technology to launch high volume and more sophisticated attacks.
Banks have a longer breath to sit out the AI/ML innovation phase using their strong balance sheets, which gives them a strategic advantage. This is significant in the context of AI because it allows financial institutions to look at AI as a portfolio of investments, not every single initiative needs to hit the mark as long as the portfolio return is optimised. This should deliver them success in the long run based on their ability to fund more projects, let some fail, and extract superior returns from scaling the best ones.
The Challenge
The hype around Generative AI as an growth accelerant has raised awareness of data platforms at the executive and board level. However, their defensive posture is primarily concerned with ensuring no data leaks occur. There is still a relatively low level of awareness about reputational and litigation risks posed by AI if, for example, they launch a biassed chatbot or credit engine that is using race or gender as a discriminating factor.
Therefore, responsible and ethical AI is a priority. This is not about removing all biases from processes like loan applications, it is about ethically justifiable and acceptable biases for specific circumstances.
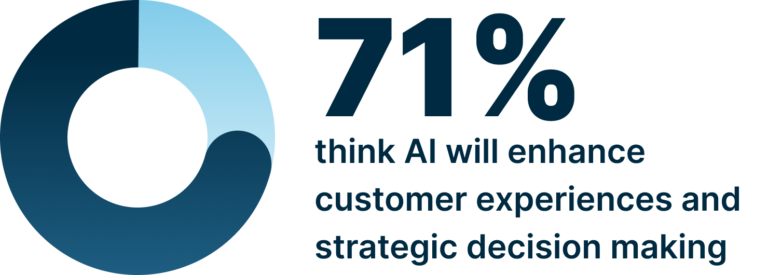
4. Democratisation of AI/ML across the entire organisation through low or no-code platforms
Some modern AI and ML platforms negate the need to be a software developer, or to know how to write code, allowing financial services professionals to embed it in every key process, and every business unit. It removes the need for a centralised AI/ML team to run and maintain all ML models. In time, this will give rise to large operational transformations, however we have seen very few to date.
Extracting the most out of AI and ML in a coordinated manner (rather than organically or incidentally in pockets of the organisation) requires upskilling your entire workforce in hands-on AI/ML skills.
The Challenge
Public professions around AI adoption have some parallels with companies’ climate commitments from five years ago. We are in a phase where public pronouncements are followed by a period of fast peddling internally to catch up and match up.
Basic AI awareness training will be superseded by organisation-wide shifts towards a data and AI centric culture as financial services institutions move up the maturity curve.
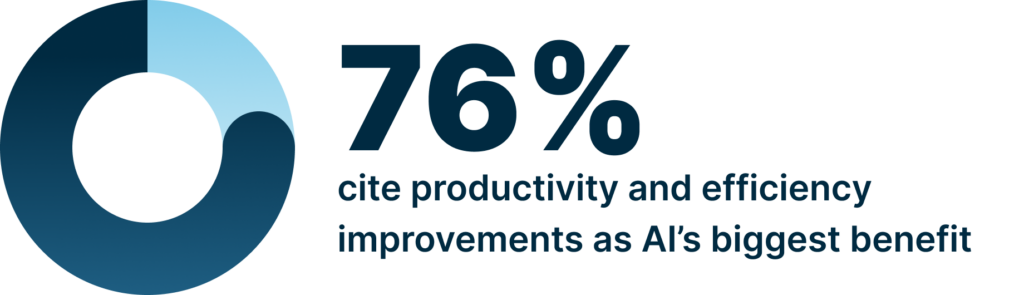