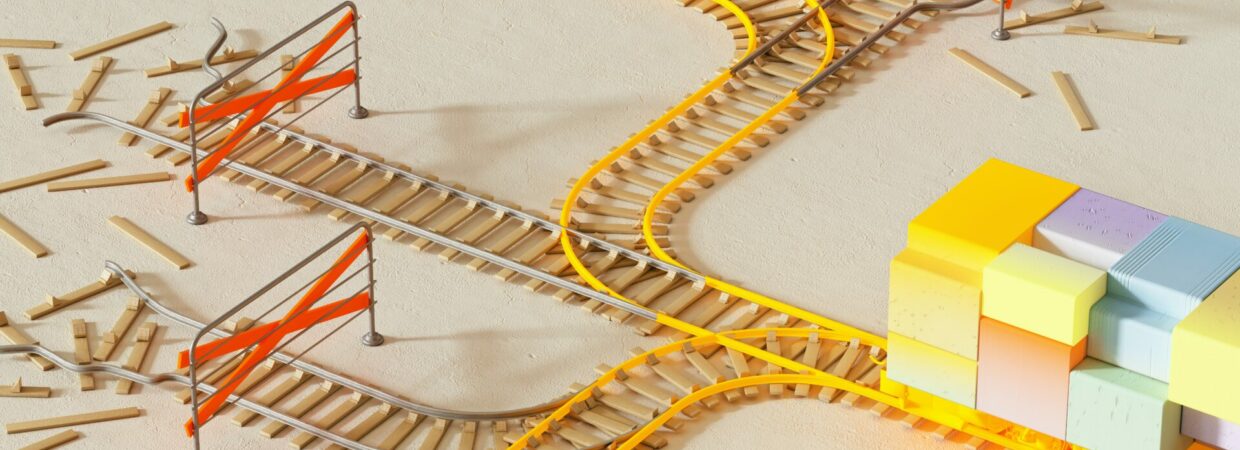
What is “Basic Analytics”?
Basic analytics, most commonly just referred to as analytics, focuses on understanding historical data. This understanding is achieved by extracting valuable insights from aggregated, clean datasets. In basic analytics, data is collected from various sources, cleaned, and transformed into a format suitable for analysis.
Data aggregation is a key component of basic analytics. It involves collecting, organising, and combining data from various sources to create a comprehensive and meaningful dataset for analysis. This process ensures that data is consistent, accurate, and ready for further examination.
The process of collecting and organising data from multiple sources is followed by data summarisation. Data summarising involves presenting a condensed version of the data, making it easier to understand. This includes calculating averages, medians, standard deviations, and other descriptive statistics that highlight key aspects of the data.
Data visualisation techniques, such as bar charts, pie charts, and line graphs, allow a more comprehensive view of descriptive statistics than just data summarisation alone. Data visualisation techniques represent data graphically, allowing users to quickly grasp trends and relationships within the data. Data visualisation techniques are commonly employed across various business domains, including sales, marketing, finance, and operations.
Monitoring KPIs, identifying areas of improvement, and tracking the effectiveness of business strategies are some uses of basic analytics that commonly have a disproportionate Return on Investment (ROI). For instance, website traffic analysis, social media engagement monitoring, sales performance tracking, and marketing campaign evaluation all benefit from basic analytics. However, basic analytics does not provide in-depth information about the causes of observed trends or predictions about future performance.
Advanced analytics techniques, such as predictive analytics and prescriptive analytics, help provide in-depth information. By identifying causal relationships and generating forecasts, these techniques support more complex decision-making processes through being future-oriented. Despite not offering the same depth of analysis, basic analytics is an essential starting point for organisations seeking to leverage data-driven insights for growth and optimised operations.
Benefits of analytics
Basic analytics offer numerous advantages for businesses, especially for those beginning to embrace data-driven decision-making. Data-driven decision-making (DDDM) is a process where decisions are based on the analysis of data, rather than relying solely on intuition or experience.
By adopting basic analytics, organisations can take their first steps toward leveraging data to gain insights, identify trends, and make more informed decisions. This approach enables businesses to improve efficiency, reduce risks, and optimise resource allocation, ultimately leading to better overall performance. Furthermore, basic analytics provide a foundation for companies to gradually build their data capabilities and evolve toward more advanced analytics techniques, ensuring they remain competitive
These insights empower companies to make informed decisions that positively impact their bottom line. In this section, we will explore the primary benefits of basic analytics, including ease of use, cost-effectiveness, the ability to identify trends and patterns, and enhanced decision-making capabilities.
Ease of use
One of the most significant benefits of basic analytics is their user-friendly nature. The user-friendly nature of basic analytics platforms comes from intuitive interfaces that simplify data access, navigation, and interpretation for even non-technical users.
Rapid value generation
This ease of use allows businesses to quickly gain insights and make data-driven decisions without investing in extensive training or hiring dedicated data analysts. The reduced need for training or hiring data analysts is a key advantage of basic analytics, as it enables users from various departments to contribute to data-driven initiatives.
Cost-effectiveness
Basic analytics tools are generally more affordable compared to advanced analytics solutions, making them an attractive option for small and medium-sized businesses or those with limited budgets.
As a result, companies can make smarter decisions without putting undue strain on their financial resources. The cost savings from using basic analytics can be redirected towards other important business initiatives, such as product development or marketing efforts, ensuring a more balanced allocation of resources.
Identifying trends and patterns
While basic analytics may not offer the same depth of analysis as advanced analytics, they are still highly effective at identifying trends and patterns in data. The ability to identify trends and patterns can help businesses understand what is currently happening in the business, and build a clear picture for informed decision making.
Improved decision-making
With the insights gained from basic analytics, businesses can make better-informed decisions based on data, rather than relying on intuition or guesswork. Data-driven decision-making can lead to improved marketing strategies, more efficient operations, and ultimately, increased revenue.
For example, by analysing website traffic and user behaviour, businesses can identify popular products or services. Identifying popular products or services helps companies prioritise marketing efforts and tailor their offerings to better meet customer needs. This will result in more efficient bids for Google Adwords, greater knowledge of which products to prioritise, and an overall north star for improvement. Additionally, basic analytics can help identify bottlenecks in operations, enabling companies to streamline processes and improve overall efficiency.
Limitations of basic analytics
While basic analytics offer valuable insights and benefits for businesses, they also have certain limitations. These limitations can hinder businesses’ ability to gain deeper insights, predict future outcomes, or understand complex relationships within data. In this section, we will discuss the main limitations of basic analytics, including limited depth of analysis, lack of predictive capabilities, challenges with unstructured data, and inability to scale with growing data volume.
Lack of predictive capabilities
Basic analytical tools generally lack predictive capabilities. The absence of predictive capabilities means they are unable to forecast future trends or outcomes based on historical data. This limitation can be a significant drawback for businesses that want to anticipate customer behaviour, optimise marketing strategies, or prepare for potential risks. Without predictive capabilities, organisations may miss out on opportunities for growth or fail to address potential issues before they become critical.
Challenges with unstructured data
Another limitation of basic analytics is their difficulty in handling unstructured data, such as text, images, or audio files. Unstructured data often contains valuable insights that can provide a more comprehensive understanding of customer preferences, sentiment, or behaviour.
Basic analytics tools are primarily designed to analyse structured data. This focus on structured data means they may struggle to extract meaningful insights from unstructured data sources. The struggle with unstructured data can leave businesses with an incomplete view of their data landscape, potentially leading to suboptimal decision-making.
Inability to scale with growing data volume
As businesses grow and data volume increases, basic analytics tools may struggle to keep up with the demands of processing and analysing large amounts of data. The lack of scalability in basic analytics tools can result in slower data processing times, reduced efficiency, and limited insights.
This inability to scale can hinder businesses from fully leveraging the power of their data, particularly as they continue to expand and generate more data over time. The growing data volume emphasises the need for more advanced analytics tools that can effectively handle the increasing complexity and scale of modern data environments.
What is “Advanced Analytics”?
Advanced analytics refers to a set of sophisticated techniques and tools that enable businesses to extract deeper insights, make accurate predictions, and optimise decisions based on large and complex datasets.
Advanced analytics allows organisations to uncover hidden patterns, discover previously unknown relationships, and develop data-driven strategies that drive growth and innovation. In this section, we will discuss the key components of advanced analytics, including predictive analytics, prescriptive analytics, and machine learning, and how they contribute to a more comprehensive understanding of data.
Predictive analytics
Predictive analytics is a subset of advanced analytics that focuses on using historical data to make accurate forecasts of future events or trends. By employing statistical models and machine learning algorithms, predictive analytics identifies potential opportunities, anticipates customer behaviour, and mitigates risks. The ability to anticipate customer behaviour and mitigate risks is instrumental in optimising marketing campaigns, improving supply chain efficiency, and enhancing overall business performance.
Prescriptive analytics
Prescriptive analytics goes beyond predictive analytics by providing actionable recommendations based on data analysis. It combines optimisation techniques, simulation models, and decision analysis to help businesses determine the best course of action under various scenarios. The determination of the best course of action under various scenarios allows organisations to identify the most effective strategies, allocate resources efficiently, and make informed decisions that support their business objectives.
Machine learning
Machine learning, a subfield of artificial intelligence, is a key component of advanced analytics. Machine learning involves the development of algorithms that can learn from and make predictions or decisions based on data without explicit programming. The ability to learn from data enables machine learning to uncover complex patterns and relationships in large datasets.
Extending traditional techniques
These complex patterns and relationships can provide valuable insights that may not be possible with traditional analytics techniques. Applications of machine learning in advanced analytics include natural language processing, image recognition, and anomaly detection, among others. By incorporating machine learning into their analytics strategy, businesses can unlock the full potential of their data and drive innovation across various domains.
Advanced analytics tools and techniques
Advanced analytics tools are essential for businesses to unlock deep insights, make accurate predictions, and optimise decision-making. These tools often incorporate cutting-edge technologies, such as machine learning, artificial intelligence, and big data processing. In this section, we discuss popular advanced analytics tools, including data mining tools, big data analytics platforms, and artificial intelligence-based solutions, along with their subcategories and specific applications.
Data mining tools
Data mining tools extract valuable information from large datasets by identifying patterns, relationships, and trends. These patterns and relationships can inform decision-making and drive business growth.
Clustering and classification tools
Clustering and classification tools group similar data points and categorise them based on shared characteristics. Examples of such tools include Weka, scikit-learn, and TensorFlow, which provide various algorithms for effective data organisation and categorization.
Association rule mining tools
Association rule mining tools identify relationships between variables and help uncover hidden patterns within data. Tools like Apriori, Eclat, and FP-Growth are widely used for this purpose, enabling businesses to find correlations that can inform strategic decisions.
Big data analytics platforms
Big data analytics platforms handle the processing and analysing of massive data volumes that traditional analytics tools may struggle to manage. These platforms, such as Apache Hadoop, Apache Spark, and Google BigQuery, provide distributed computing capabilities, allowing businesses to scale their analytics efforts as data needs grow.
Data storage and management solutions
Data storage and management solutions, like Hadoop Distributed File System (HDFS) and Amazon S3, ensure efficient storage, retrieval, and processing of large datasets. They provide the foundation for big data analytics platforms to operate smoothly.
Data processing frameworks
Data processing frameworks, such as Apache Spark, Flink, and Beam, enable efficient data manipulation and transformation for big data analytics. They provide powerful processing and analytical capabilities, handling both batch and real-time data processing.
Artificial Intelligence-based solutions
Artificial intelligence (AI) plays a significant role in advanced analytics by automating complex data analysis processes and deriving insights more efficiently. AI-based solutions like IBM Watson Analytics, Google Cloud AI Platform, and Microsoft Azure Machine Learning incorporate machine learning algorithms, natural language processing, and computer vision techniques for in-depth analysis and predictions.
Natural language processing tools
Natural language processing (NLP) tools, such as spaCy, NLTK, and Gensim, facilitate the analysis of textual data by extracting meaningful insights and patterns. They enable sentiment analysis, topic modelling, and entity recognition, among other applications.
Computer vision tools
Computer vision tools, like OpenCV, TensorFlow, and Caffe, empower businesses to analyse and interpret visual data, such as images and videos. These tools help with object recognition, image segmentation, and facial recognition, providing valuable insights for various industries.
Benefits of advanced analytics
Advanced analytics offers numerous benefits to businesses, allowing them to uncover deeper insights, make more accurate predictions, and optimise decision-making processes. By harnessing the power of advanced analytics, organisations can gain a competitive edge and drive growth. In this section, we will discuss the key benefits of advanced analytics, including improved decision-making, enhanced customer experience, increased efficiency, more accurate forecasting, optimised marketing strategies, risk management, innovation, and better talent management.
Improved decision-making
One of the primary benefits of advanced analytics is its ability to support data-driven decision-making. By leveraging advanced analytics techniques such as machine learning, artificial intelligence, and big data processing, businesses can uncover hidden patterns, trends, and relationships within their data. These insights enable organisations to make more informed decisions, optimise business strategies, and identify new opportunities for growth. Additionally, advanced analytics can help businesses recognise potential problems and develop preventive measures, thus avoiding costly mistakes.
Enhanced customer experience
Advanced analytics can help businesses improve their customer experience by providing a deeper understanding of customer behaviour and preferences. By analysing customer data and uncovering patterns, organisations can tailor their products, services, and marketing efforts to better meet customer needs. This personalised approach can lead to increased customer satisfaction, loyalty, and long-term revenue growth. Moreover, advanced analytics enables businesses to identify and address customer pain points, further enhancing the overall customer experience.
Increased efficiency
Advanced analytics brings increased efficiency to various business processes. Through automation and the use of artificial intelligence, organisations can streamline their operations, reduce manual tasks, and minimise the risk of human error. This increased efficiency can lead to cost savings, improved resource allocation, and higher overall productivity. Furthermore, advanced analytics can help businesses optimise their supply chain management, inventory control, and workforce scheduling, resulting in more efficient operations.
More accurate forecasting
Advanced analytics enables businesses to make more accurate forecasts and predictions by leveraging advanced techniques like machine learning and predictive modelling. These methods can help organisations identify trends, anticipate future events, and make proactive decisions to stay ahead of the competition. More accurate forecasting can lead to better resource planning, risk management, and strategic decision-making. It also allows businesses to capitalise on emerging market opportunities and adapt to changing customer demands more effectively.
Optimised marketing strategies
Advanced analytics allows businesses to optimise their marketing strategies by better understanding customer behaviour, preferences, and trends. By analysing large volumes of data, businesses can identify the most effective marketing channels, target audiences, and promotional strategies, leading to higher return on investment (ROI) and greater overall marketing success. Additionally, advanced analytics can help organisations measure the performance of their marketing campaigns, enabling them to make data-driven adjustments for maximum impact.
Risk management
By leveraging advanced analytics, organisations can better assess and manage risks. Advanced analytics techniques can help businesses identify potential threats, monitor emerging trends, and implement proactive measures to mitigate risks. Improved risk management can protect an organisation’s assets, reputation, and long-term viability. Advanced analytics can also help businesses maintain regulatory compliance, reducing the likelihood of costly fines and penalties.
Driving innovation
Advanced analytics can spur innovation within an organisation by uncovering new opportunities, identifying market gaps, and providing insights into customer preferences. By analysing large data sets, businesses can discover novel ways to solve problems, create new products or services, and enhance existing offerings. This innovation can lead to a competitive advantage and contribute to long-term business success.
Better talent management
Advanced analytics can help organisations optimise their talent management processes by providing insights into workforce trends, employee performance, and skill gaps. By analysing employee data, businesses can make data-driven decisions related to hiring, training, performance management, and workforce planning. This can lead to improved employee engagement, reduced turnover, and a more efficient and effective workforce.
Challenges of advanced analytics
Advanced analytics, while offering numerous benefits, also presents challenges that organisations must navigate. These challenges include data quality and management, skill gaps and talent acquisition, complexity and integration, cost considerations, data privacy and security, and maintaining stakeholder trust. In this section, we will explore each challenge in greater detail, providing insights into how organisations can address them effectively.
Data quality and management
The success of advanced analytics initiatives is heavily influenced by data quality, as poor data quality can lead to inaccurate or misleading insights. To ensure data quality, organisations must invest in data cleansing, validation, and standardisation processes. These processes help maintain data integrity, which is crucial for deriving valuable insights from advanced analytics. In addition, data management practices such as data governance and data architecture are essential for preserving data quality and facilitating the smooth flow of data throughout the organisation.
Skill gaps and talent acquisition
To implement advanced analytics, organisations require specialised skills, including expertise in data science, machine learning, and artificial intelligence. However, many organisations face a talent gap, as professionals with these skills can be difficult to find and recruit. To bridge this gap, businesses can invest in employee training and development programs, establish partnerships with educational institutions, and consider outsourcing or hiring consultants with the necessary expertise.
Complexity and integration
Advanced analytics techniques and tools can be complex, particularly for businesses with limited technical expertise. This complexity extends to integrating these advanced tools with existing systems and processes. To tackle this challenge, organisations need to invest time and resources in understanding the technologies, developing a cohesive analytics strategy, and ensuring seamless integration with their current infrastructure.
Cost considerations
The costs associated with implementing advanced analytics can be significant, particularly for small and medium-sized businesses. These costs include the price of advanced analytics tools, hiring skilled professionals, and investing in data management and infrastructure. To ensure cost-effectiveness, organisations must carefully evaluate the potential return on investment (ROI) and assess the long-term benefits of advanced analytics initiatives.
Data privacy and security
As organisations collect, process, and analyse large volumes of sensitive data, data privacy and security become major concerns. Compliance with data protection regulations and ensuring data security are essential for maintaining customer trust and avoiding legal and financial consequences. Implementing robust security measures and adhering to data privacy best practices help organisations address these concerns effectively.
Maintaining stakeholder trust
The use of advanced analytics can raise concerns among stakeholders, particularly with regards to data privacy and potential biases in decision-making. To maintain stakeholder trust, organisations must be transparent about their data practices and address potential biases in their algorithms. Furthermore, clear communication of the benefits of advanced analytics initiatives is vital for securing stakeholder support.
Basic vs. advanced analytics
When comparing basic and advanced analytics, it is essential to understand the key differences between the two approaches. These differences can impact various aspects, such as the purpose of the analysis, techniques used, required skill sets, and implementation costs.
The following table provides a clear and concise comparison of basic and advanced analytics, highlighting their main characteristics and how they differ from one another;
Aspect | Basic Analytics | Advanced Analytics |
Purpose
|
Analysing historical data to understand trends and patterns | Using complex algorithms to predict future trends and behaviours |
Techniques
|
Descriptive statistics, data visualisation, and reporting | Machine learning, data mining, statistical modelling, AI |
Data requirements
|
Structured data, smaller datasets | Structured and unstructured data, large datasets |
Focus
|
What has happened in the past | What will happen in the future or what should happen next |
Skill set required
|
Basic statistical knowledge, data manipulation skills | Data science, machine learning, AI expertise |
Tools
|
Spreadsheets, basic reporting tools, simple BI tools | Advanced BI tools, machine learning frameworks, big data tools |
Complexity
|
Relatively simple and straightforward | Complex, often requiring specialised skills and knowledge |
Use cases
|
Performance tracking, KPI measurement, summarising data | Forecasting, sentiment analysis, customer segmentation, anomaly detection |
Implementation cost
|
Generally lower due to simpler tools and techniques | Higher due to the need for specialised tools, talent, and infrastructure |
Time to insight
|
Faster, as it deals with simpler analysis and smaller datasets | Slower, as it involves complex algorithms and larger datasets |
Data management and quality
|
Less demanding, as it primarily deals with structured data | More demanding, as it requires data governance and data cleansing |
The table above offers a comprehensive comparison of basic and advanced analytics, showcasing the primary distinctions between the two. While basic analytics focuses on understanding historical data and identifying patterns, advanced analytics delves deeper, utilising complex algorithms and techniques to predict future trends and behaviours.
Ultimately, the choice between basic and advanced analytics depends on your organisation’s needs, resources, and objectives. By understanding these differences, you can make informed decisions about which approach is best suited for your specific business context and goals.
Which analytics approach is right for your business?
Selecting the most appropriate analytics approach for your business is crucial for successful data-driven decision-making. The right choice hinges on multiple factors, including business goals, available resources, and organisational maturity in data-driven decision-making. In this section, we will delve into these factors and explore key considerations in greater detail to guide you in deciding between basic analytics or advanced analytics for your organisation.
Assess your business objectives
Determining the right analytics approach begins with identifying your organisation’s primary goals and objectives in data analysis. Business goals play a pivotal role in this decision, as basic analytics is tailored for businesses seeking performance tracking, KPI measurement, and historical data insights. Conversely, advanced analytics caters to organisations aiming for data-driven predictions, pattern identification, and complex data insights. Depending on your goals, either basic or advanced analytics will be more suitable for your needs.
Evaluate your data infrastructure and resources
Data infrastructure and resources are also key factors in deciding the appropriate analytics approach. Assessing your organisation’s existing data storage, processing capabilities, and available analytical tools is essential. For organisations with limited resources, a smaller IT team, or lacking data infrastructure, basic analytics may be the better choice. However, if your business has access to advanced tools, technology, and skilled data scientists, advanced analytics can provide deeper insights and drive more informed decision-making.
Consider the complexity of your data
The complexity and size of your data significantly impact which analytics approach best suits your organisation. Basic analytics typically works well with structured data and smaller datasets, making it ideal for organisations dealing with well-defined data sources and straightforward analytical questions. Advanced analytics, on the other hand, can handle both structured and unstructured data, as well as larger and more complex datasets. This approach is particularly beneficial for organisations with diverse data sources and large data volumes.
Determine your organisation’s analytics maturity
Your organisation’s current analytics maturity level is another essential aspect to consider when choosing an analytics approach. Assessing your business’s data-driven decision-making, employee skills, and data management processes will help you gauge your analytics maturity. Starting with basic analytics can establish a solid foundation and create a data-driven culture for businesses new to data analysis. In contrast, organisations with data analysis experience can enhance their analytical capabilities with advanced analytics, despite the potential need for a greater investment in technology and personnel.
Quick plug – we literally do these kinds of projects every single day, and have worked with some of the largest brands in Australia. Feel free to reach out for a no-obligation chat with our team of friendly experts.