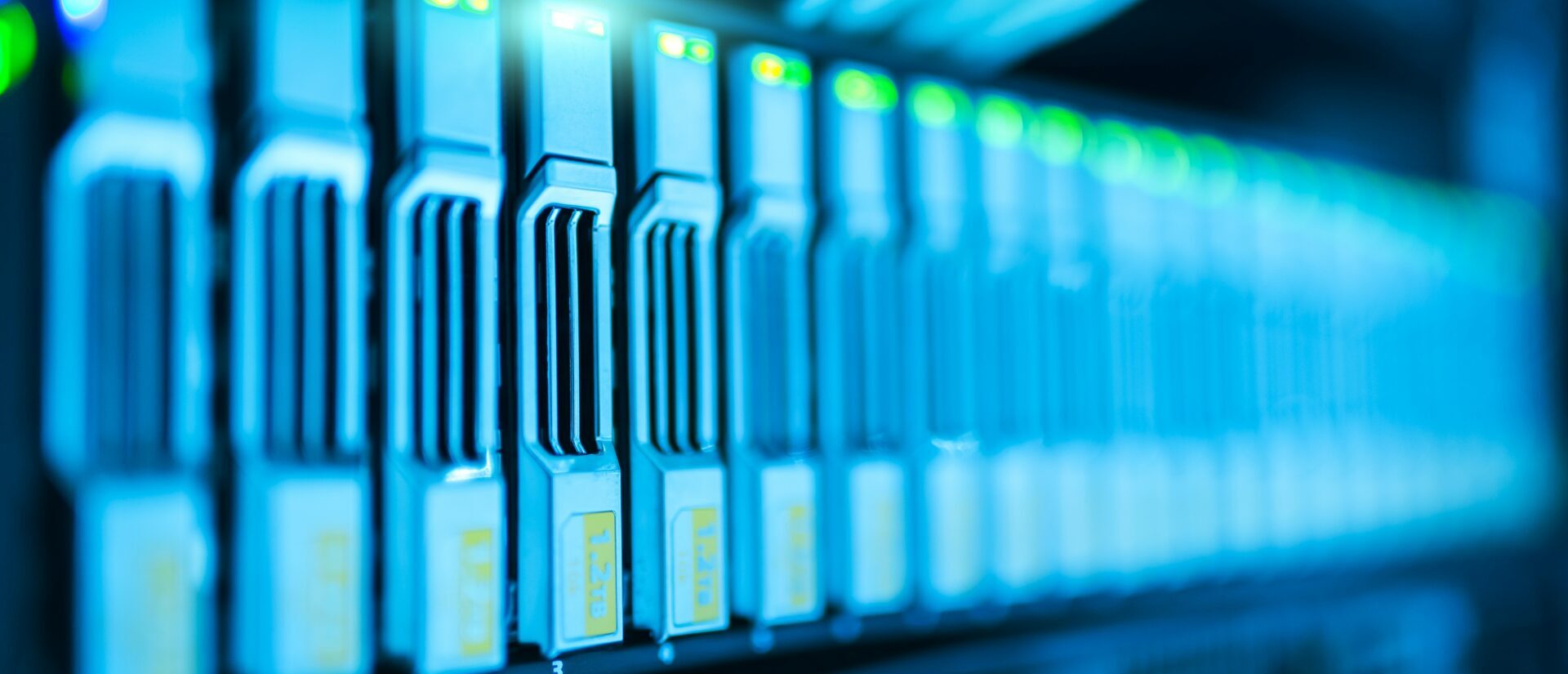
What is big data?
Big data refers to large, complex data sets often contrived from multiple and new sources. The reason that “big data” has received its own term (after all, it really just means larger datasets) is due to the added complexity that comes with simply adding more data, particularly from multiple sources.
Traditional data models and processing can’t manage the exponentially increasing volume that is big data, creating a need for more specialised tools such as Hadoop and more recently, Spark. These added complexities make big data engineering a rarer and more specialised skill than traditional data engineering.
So what’s the solution?
It starts with gaining an understanding of the issue. As more and more data is produced and collected, the world needs ways to organise and describe it. This is where the five Vs can assist with conceptualising and utilising big data in the context of your business.
The five Vs of big data
The five Vs of big data (volume, velocity, variety, veracity and value) are like the five Ws of Journalism (who, what, why, where and when). They’re the characteristics that define big data and what data analysts, engineers and executives need to understand when considering their organisation’s approach to data.
Volume
Volume refers to the size (per unit, i.e., one terabyte) and quantity (number of units, i.e., one million records) of data. How big and how much will vary widely depending on industry, organisation and technological advances over time.
For example, a dataset that is considered big data today may not be considered big data in 5 years, as computing power and what constitutes ‘large data volumes’ is evolving at a rapid pace. In the past couple of decades, there has been an exponential increase in the volume of data that organisations are able to capture. This is the foundation of why big data exists and leads us to the remaining four Vs.
Examples
- A standard financial services enterprise handles more than 1.5 million transactions every hour, which imports over 2.5 petabytes into their database. For comparison, that’s like having over 40 million filing cabinets filled with text.
- Thanks to advances in technology such as smartphones and wearable digital devices like watches, it’s estimated that over 30% of the world’s data is generated by healthcare companies and will reach nearly 5,000 device interactions per day by 2025.
- On a national level, educational institutions collect and store data of millions of students, including grades, test scores, expulsion records, extracurricular activities and more to share with universities for admissions considerations.
Velocity
Velocity is the speed that companies receive, store and manage the data coming in from its various data sources. It’s important because some data will be far more valuable the closer it is to instantaneous.
Consider the world of retail. It’s way better to know which products are out-of-stock in terms of seconds or minutes rather than days or weeks. Telling a customer to come back tomorrow to find out whether you have a particular shoe in stock isn’t exactly a great sales tactic.
Examples
- In the finance sector, an investment company uses big data to pull trends from multiple sources fast enough to provide real-time stock market insights to help investors make informed decisions with their money.
- A medical device company receives heart rate data from a pacemaker and needs to process it and produce rapid analysis to provide a reactive shock to restore heart rhythm, preventing serious injury or death.
- A large university opens enrolment for the semester for tens of thousands of students. The university must process this information instantaneously to provide accurate information as students continue to register for classes until capacity is reached.
Variety
One of the challenges that accompanies the creation of big data infrastructure is all the different sources upstream that are flowing into the data lake. Variety of big data refers to the diversity and range of different data types, including unstructured data, semi-structured data and structured data, along with their disparate sources.
Examples
- A national bank collects structured data from forms on their website and mobile app, where each field maps directly to a table in their database. However, they also collect unstructured data from customer service phone calls and emails.
- About 80% of healthcare data is unstructured, including handwritten practitioner notes, imaging data, video and audio files and many more. Many healthcare companies are adopting EHRs (electronic healthcare records) that help structure some incoming data, but the challenge of transforming unstructured big data still exists.
- A university collects semi-structured data on individuals in a student profile through academic transcripts, purchases made with linked credit cards and admissions to different facilities used with their student ID card.
Veracity
How trustworthy is the data? Veracity in big data can be thought of as its credibility or reliability. If users don’t believe in the data or have too many doubts about its validity or quality, the data loses value and becomes irrelevant, misleading or dangerous. Given the movement of organisations toward data-driven decision-making, weaknesses in data veracity will ultimately impact the choices that executives make.
One of the biggest obstacles to organisations becoming data-driven is their lack of best practices around data governance. Fortunately, ensuring the quality of data at your organisation is something relatively simple, and really just comes down to following a few steps.
Examples
- A financial firm that allows customers to enter their address without verifying that the address actually exists is more likely to have human errors as people could lie or enter typos, lowering veracity.
- A healthcare company has discovered that multiple patients have the same Patient ID due to historic sources being consolidated into a new, modern data platform.
- An educational institution wishes to analyse academic performance amongst all primary school aged children, but different grading scales and standards are used at different schools, making the data unreliable.
Value
From a business perspective, value is the most important characteristic of big data. Value is derived from the analysis, insights, discoveries, and ultimately business decisions and consumer outcomes that result from the data collected.
Determining the value of data requires working backwards to determine what decisions are now viable as a result of the new information, and the expected economic utility of those decisions.
A good way to ensure that useful data is being collected comes from identifying areas where you are lacking information and using that as a basis for exploratory analysis.
Big data analytics
Big data analytics revolves around extracting insights from large bodies of information. Thanks to Big Data analytics, Google Maps can give you the optimal route to any destination, taking into account traffic, weather conditions, road closures and a multitude of other factors that Google collects in its vast datasets.
Organisations that leverage big data will be leaders in the global economy in years to come, regardless of industry. Research shows that companies can save billions of dollars by cutting costs and implementing more efficient processes. By capitalising on consumer insights that big data can provide, the sky’s the limit on how profitable organisations can become. There are philanthropic impacts that big data will have on the world, too.
For example, in the financial services industry, leading organisations use big data analytics to crack down on fraud and money laundering as well as improve compliance with the many laws around conduct, reporting and corporate transparency. This will not only benefit financial institutions, but also society as a whole as dishonest behaviour will be caught and decrease over time.
In the healthcare sector, companies can gain profitability but also help save lives by analysing trends and threats in patterns of data. Big data will have a huge role in the future of genomic research, patient experience, claims and billing fraud and more.
Organisations need skilled people effectively utilising the latest data engineering technology to keep up with the rise of big data and benefit from the value analytics provide. We get it – data projects can be difficult to actualise within an organisation, especially when internal stakeholder approval is needed. Aginic hires and trains individuals who are experts in engineering and data, capable of creating complete end-to-end data solutions that help create lasting change.