Vihan Patel, October 2024
AI Factories should be able to lift the volume, success, value, and speed of AI innovation within organisations.
CommBank’s decision to set up an AI Factory with Amazon Web Services to accelerate Gen AI innovation has sparked debate about what AI Factories are, who needs one, and why now?
In establishing several AI Factories for large ASX-listed entities we have found different variants, each with a slightly different priority.
Some are an attempt to put guardrails around the massive amount of innovation occurring in this field, often under the radar and outside normal governance frameworks. These suit large enterprises with often siloed and federated technology operating models.
Others aim to accelerate the innovation process and/or clear a backlog of AI use cases to extract value faster. These tend to suit smaller, nimble organisations where a single AI roadmap can be centrally managed.
Shoring up access to valuable computational resources, particularly in regions like Australia, also underpins many of the AI Factory collaborations with tech majors like AWS, Dell, Google, Microsoft, and Nvidia.
Generative AI models consume an enormous amount of compute resources and there is growing sense it may become a scarce commodity impacting competitive advantage.
Regardless of the intent, AI Factories should aim to lift the volume, success, value, and speed of AI innovation within organisations by implementing uniform processes.
Why do we need AI Factories?
AI Factories can accelerate experimentation by connecting useful data and solution templates. They can focus on rapid prototyping sprints to fail fast or scale use cases quickly. Or, they may build and support core services shared by AI solutions teams across the organisation in a ‘hub-and-spoke’ model.
In essence, AI Factories have superseded the old innovation hubs, landing zones, and ‘innersourced’ code repositories which helped organisations navigate the last decade of digital disruption and adoption of cloud services.
This might just sound like a simple rebranding exercise, but it’s not.
AI Factories signify a paradigm shift in thinking about AI and how an organisation uses it to propel future growth.
For boards and CEOs, success and value realisation of AI initiatives has a direct correlation to quality and quantity of initial ideas entering their AI innovation funnel.
With more investment in AI comes greater scrutiny and expectations around shareholder value creation.
The value of AI will ultimately be determined by the volume of use cases going into the funnel, the number of successful experiments exiting it, their total value, and their speed through the funnel. An AI Factory should be able to improve all of those factors.
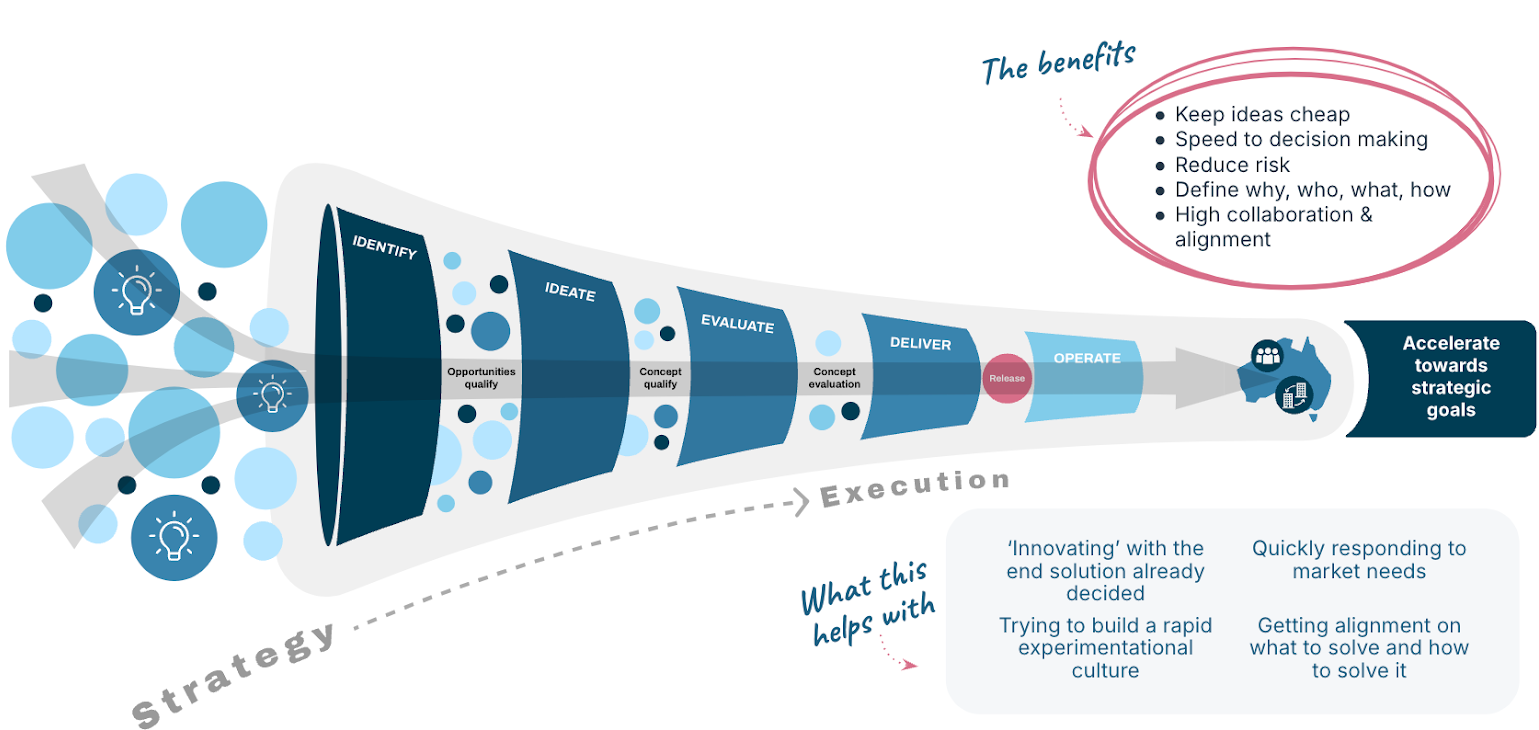
At the same time, a dramatic shift in where AI innovation is occurring within organisations is creating a sense of urgency.
Ten years ago, responsibility for AI sat comfortably within the data science and analytics team under the watchful eye of the chief technology officer. They identified use cases, experimented to make the business case, then built AI applications for business value. This central function is evolving with the focus shifting towards internal AI education and enablement to remove bottlenecks in AI innovation.
Today, AI has been democratised and touches every process in every part of the business. Everyone wants to do it, and everyone can do it because with the advent of Large Language Models and models-as-a-service you don’t need data scientists to work with AI. Any software engineer can build a Gen AI chat bot, opening up massive opportunity but also exposing an organisation to massive risk.
To combat this combination of unskilled practitioners and powerful models, regulators have tightened compliance in an effort to govern AI more closely.
Questions for business leaders:
- What is the best operating model for AI in the organisation?
- What elements should be centralised and which should be federated, if any?
- How do we want to govern this operating model?
- How do we enable the rest of the organisation to safely and successfully experiment with and deliver value with AI?
- How do we avoid cost wastage from repeated or low-value initiatives?
- How do we manage risk and ensure experiments that won’t pass the risk/legal/compliance check are divested early?
- How do we establish the right kind of team structure / skillset to support AI innovation in what is already a tight market for AI talent?