What is a data strategy?
A data strategy sets a clear objective of what companies ultimately want to achieve with data in their organisation. Most companies believe that making use of their data revolves around obtaining the newest technology in the data world. However whilst technology is important, that is only one piece of the puzzle. The best data strategy considers important factors such as what data to work with, who is using the data, how they are using it and what for. The right data strategy accompanied by a well-tailored roadmap will help companies achieve high value business outcomes in the short and long term.
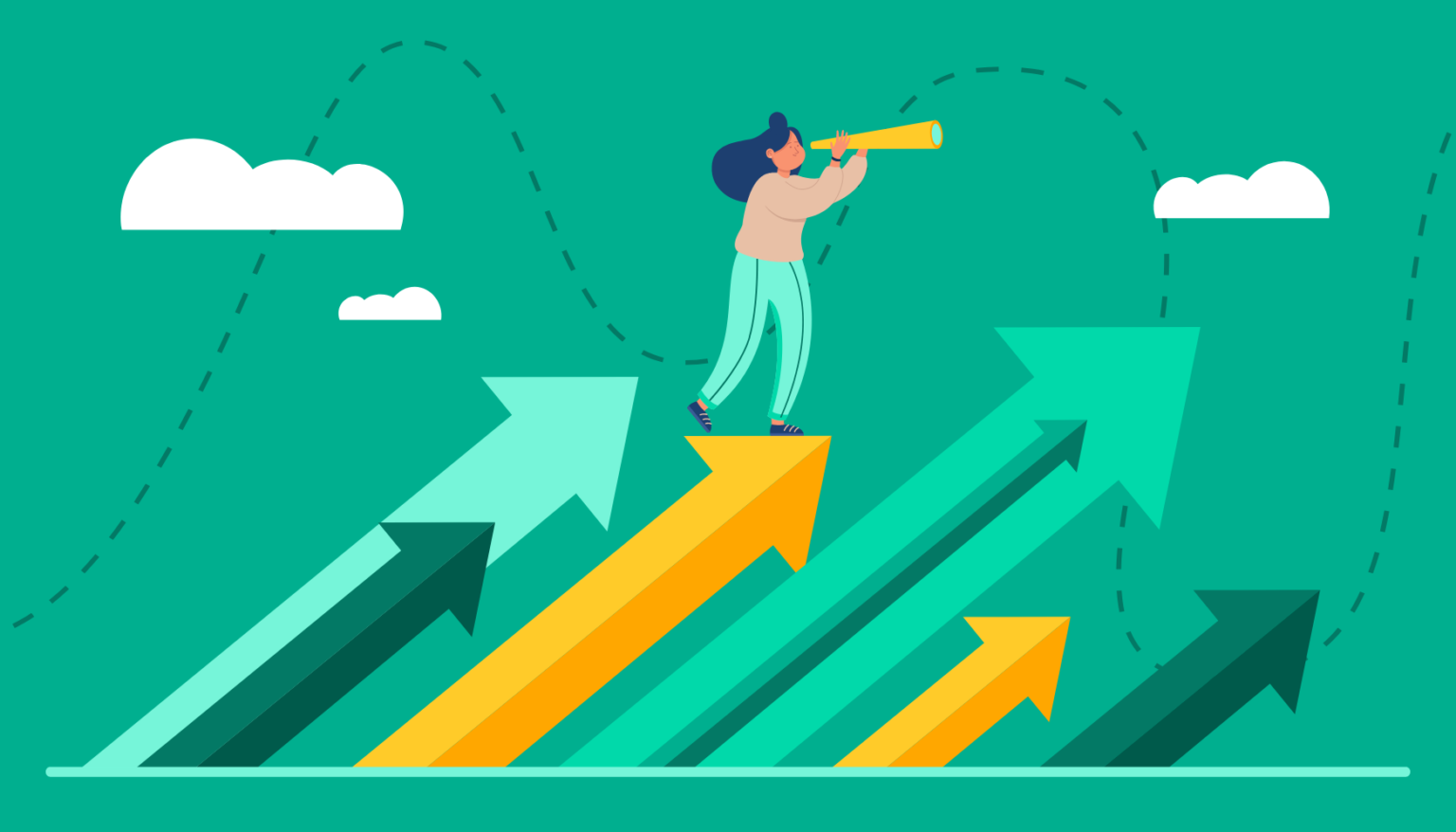
Having a clear data strategy can keep an organisation on track and focused on bigger strategic enterprise goals
What to focus on when it comes to data strategy?
In most organisations, data is scattered in silos, hidden amongst divisions and teams. But with data being at the core of every business and decision-making process, how can organisations unlock the full potential of data, become truly data-driven and embed it into day-to-day operations and strategic initiatives?
The Global Management Report found that 65% of Australian companies are having difficulties turning their data into useful insights.
Why? Most companies believe that making use of their data revolves around obtaining the newest technology in the data world. However whilst technology is important, that is only one piece of the puzzle. Simply choosing the latest technology does not mean that the technology will solve the problems a company is seeking to solve to enable their future initiatives and unlock most value from their data. As we know, it’s not just the car that makes a Formula 1 champion.
What makes a good data strategy?
The best data strategy considers important factors such as how the data is being captured or whether a company is even capturing the right data in the first place. Does the company have the right people and data culture to deal with the different layers in the data journey? Or does it have the right governance controls in place to manage data access, auditing and integrity? There are often heavy fines associated with non-compliance and even a larger reputational risk is at stake – for example, Facebook (now, Meta) is having to deal with new data laws in the EU that may mean it will no longer operate in Europe (as Meta has threatened).
Another key factor that forms a good data strategy and roadmap is socialising it with the people in the organisation and making sure that they are onboard and understand the strategy. Too often, a state of the art data lake or data warehouse is built in splendid isolation. The result is a beautiful castle that has no residents, as the rest of the organisation sometimes doesn’t even know of its existence, let alone of its beauty and capability. Or, users end up misusing the solutions – imagine the castle residents used the castle to store water instead. Imagine the panic when the water starts pouring out of the windows!!!
With all these needs around data comes the burden of managing the flow correctly to ensure reliable data quality, secure and risk-reduced data sharing and a straightforward method of communicating the data as well as its usability from the end user’s point of view.
So, what are the key elements of a data strategy?
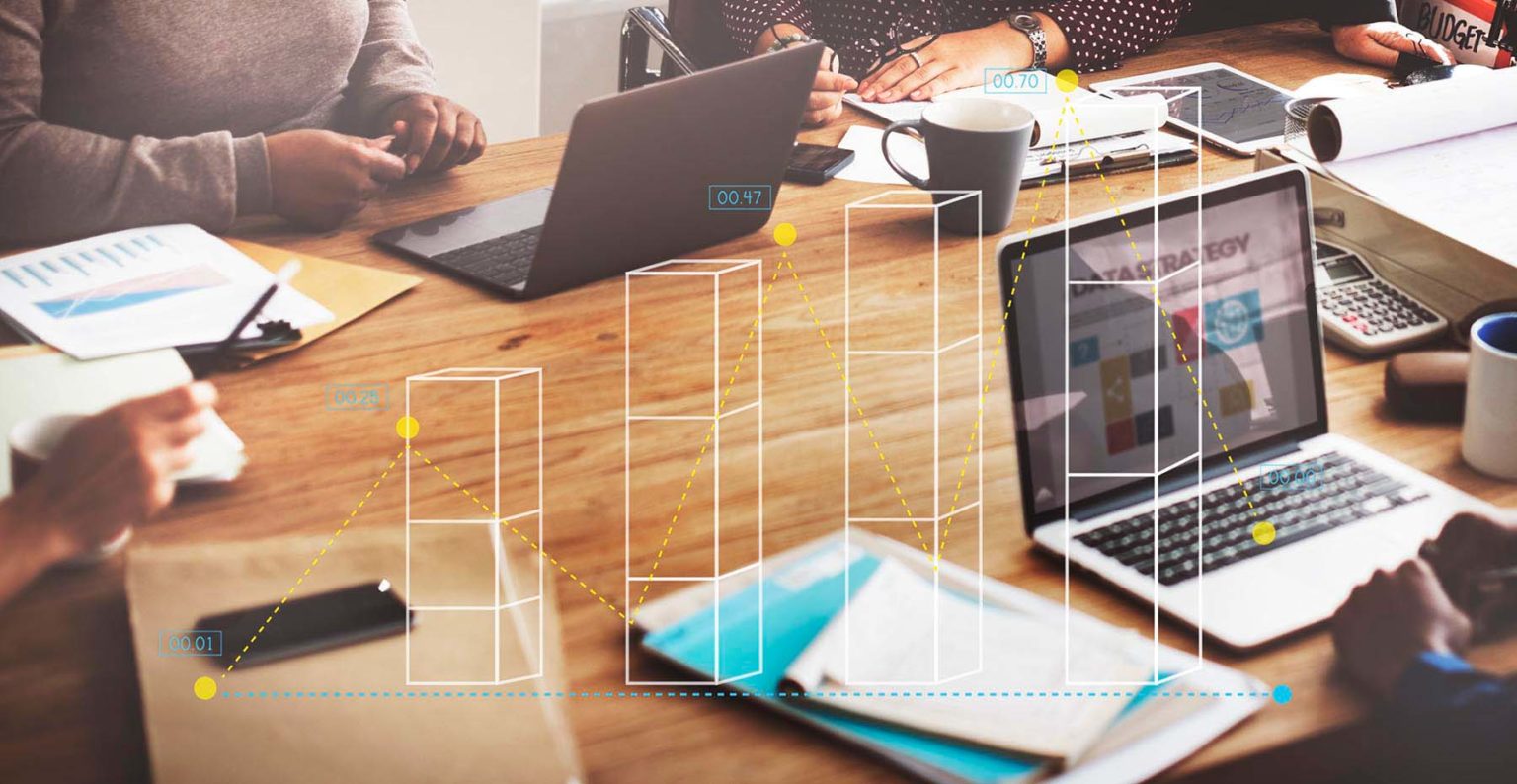
As mentioned previously, data strategy is more than a technology roadmap – it is every organisation’s playbook to utilise data effectively. It’s not just a guide to identify what technology to implement but it sets out the organisation’s strategic goals, frameworks and use cases for data. But is it really necessary to have one?
There’s more to it than just technology
Like today’s modern era of virtual meetings, when someone is on mute or when someone randomly drops off or even when someone can’t share their screen – people typically blame it on technology. Organisations also have the same mindset and are quick to put the blame on technology and systems when something goes wrong. When this happens, the immediate action is to replace it with the latest “best in class” solution. For example, a data warehouse may not be performing as well as the organisation expects it to be – but is it really the technology? Does it really have to be replaced? Or is it because the organisation is not equipped with the right data capabilities to ensure it is set up accordingly? It could also be because there are no data management practises in place, data governance frameworks are non-existent and there may even be a lack of data capabilities such as appropriate data tools to support it or data specialists to manage it.
A data strategy should enable organisations to promote and champion the power of data
Data is not just a part of technology as it has a significant impact on every inch of the business whether it’s the finance department, marketing team or the products team – data is produced and leveraged everywhere. Developing a data strategy that identifies and establishes the appropriate controls, use cases, governance framework, practices and infrastructure for data, as well as taking into consideration the people, process, and technology factors – makes the key elements to building a fit-for-purpose and effective data strategy. But in order to execute this accordingly, the why and the how must be identified. A data strategy should first and foremost identify why they need data – will it empower and drive the strategic business goals? If so, incorporate it with how the technology, people and process factors will enable the execution of the strategy. Ultimately a data strategy should be encouraging teams and individuals to become data-driven and become empowered to harness the opportunities that data brings from BI to AI.
A practical way is to take a use case driven approach by identifying opportunities with high value to the business through the concept of ‘Data Thinking’.
Most importantly; it is about people and ways of working
A data strategy is not just all about technology and process, it also takes into account people and ways of working.
The prime element to an effective data strategy is people
An effective data strategy needs to be built around the people. People who advocate the data strategy and proactively support the strategic initiatives cause a domino effect where the broader organisation begins to embrace a data-driven culture. Like what Waller said in HBR’s ‘10 Steps to Creating a Data-Driven Culture’, “the biggest obstacles to creating data-based businesses aren’t technical; they’re cultural”.
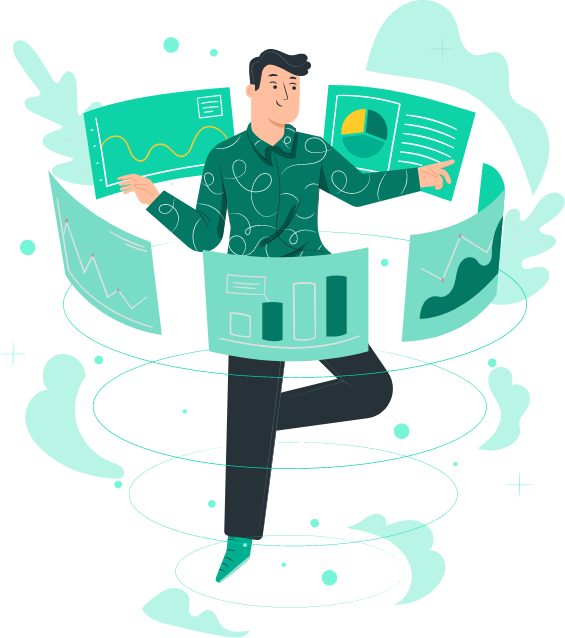
Leadership’s perception of the value of data is key
Cultural adoption of data starts with how every individual in an organisation perceives it. Being data-driven means wanting to make business decisions free from human bias. It requires the willingness to give up the liberty of making discretionary ‘gut-feel’ decisions. Typically, leading by example is the prerequisite for leadership teams to drive this cultural adoption. For example, a CEO who still relies on an excel spreadsheet to inform their weekly decisions is not setting the standards on new ways of using and consuming data. This also influences leaders and managers to adopt a similar style and affects the way data is being used and reported on from top-down in the organisation. If an organisation’s leadership team has a full grasp of the value of data, knows how it can be used and has a vision on the endless opportunities that can be made from it – this echoes down into the rest of the organisation. Without this mindset , it often leads to a tremendous lack of capabilities, whether it’s lack of resourcing (data specialists) or lack of training and materials – often organisations don’t have the right specialists or experts who can champion and share the importance and value of data.
A data-driven culture starts with having the right people
Being surrounded by data-focused leaders, data specialists and champions of data, influences the culture of the organisation to become data-driven. This then has an impact on the operating model and how people work, as embracing a data-driven culture also encourages more individuals to start using data. Whether it is by leveraging existing data use cases and build solutions, identifying ways to improve business processes to become efficient, purposely leveraging data to make business decisions, or even establishing appropriate controls and governance frameworks to ensure that data is being managed, used and defined appropriately across the business – these are just some of the examples that take place if the data strategy and the people element are combined harmoniously.
Having a data-driven culture and the right data talent puts the organisation in a position where they can better respond to the ever-changing market, swiftly address challenges, make informed decisions, identify anomalies or opportunities and stay ahead of the curve.
How to translate a strategy into a data & analytics roadmap?
Ideas without actions are just ideas. Much like strategies without a clear roadmap. If a strategy tells you why and what a roadmap tells you how. Developing a data and analytics roadmap requires understanding the business benefits (the ideas), and what actions need to be taken in order to deliver value quickly. It also requires a holistic approach where the combination of data, tech, people and processes deliver tangible business benefits. Figuring out the comprehensive set of initiatives to drive business outcomes, therefore, requires some strategic analysis.
How to balance what’s important versus what’s urgent in your roadmap
Breaking down the roadmap by setting people, process, tech and tooling on the table, it’s time to piece the puzzle together. And as with a jigsaw, one typically starts with the corner and side pieces, trying to build out the whole all at once. Correct? But wait… What if the puzzle suddenly needs to be packed away? What’s the result? Therefore, a superior tactic is to start by grouping into colours and building out parts of the puzzle. And it’s exactly the same with data! Building out the smaller pieces instead of working towards a goal that’s years in the distance will ensure that value is being delivered every step of the way even if the original longer-term picture isn’t completely achieved. And due to the increasing pace of technological change, it’s nearly certain that a long-term goal might never be achieved exactly as envisioned. The rules of the game simply change too rapidly (like the puzzle is ever-expanding).
In order to effectively develop fit-for-purpose data foundations, it should also be clear what the use case (first part of the puzzle) is. Will weekly reports suffice for decision-making purposes in the foreseeable future for this business unit? Then there is no need to facilitate streaming or real-time data.
So, what data foundations are needed to build and scale out decision-making competency?
Data management (process), data capabilities (people) and data platform and workbench (tech) are the three typical pillars to establish the base. For example, to improve data-driven decisioning, it should be a priority to ensure data is trusted, timely, available, and data flows are automated.
It is also very important to consider ensuring what is included in the data strategy is fit-for-purpose and scalable. If the technologies are selected and implemented based on market popularity rather than suitability, then the infrastructure may not be as scalable to fit the organisation’s future direction or it may be an over-engineered infrastructure that is never fully utilised and becomes an unnecessary cost to the organisation.
Having the appropriate strategic initiatives, suited for what is required now and in the future while also incorporating the right foundational pillars will ensure that an organisation’s data and analytics roadmap avoids these issues and is set up for success.
Guiding you through your data & analytics strategy and roadmap
Data & Analytics Roadmap Checklist
At Mantel Group, we use practical frameworks and tools to design a data strategy and roadmap. We take a use case driven approach to get alignment with business priorities, obtain clarity on ROI and budget, and drive faster time to value. It means we identify and prioritise high business value opportunities, scope and design clear solutions, and implement effectively. Want to know more? Have a look at our data strategy offering for clients
As everyone likes freebies, the checklist below provides practical suggestions to anyone who wants to kick-start their data strategy and D&A roadmap
Business and objectives
Key outcome: To get a clear understanding of business objectives and the current state.
- Identify business strategies, priorities, processes for improvement and objectives.
- Conduct process and maturity assessments.
- Map out how the current business processes will be impacted and define remediations to ensure that teams are supported i.e. training.
- Select the key areas of the business function to focus on.
Use case driven solution design
Key outcome: To have a high-level solution design for the prioritised use cases.
- Turn objectives and assessment outcomes into use cases and analytical requirements.
- Prioritise the use cases and identify which aligns with the overall strategy and operations.
- Develop a plan and a timeline to map how each use case will be built into solutions over time.
- Develop a high-level solution design.
- Estimate the investment required and returns.
Data capabilities
Key outcome: To establish a roadmap and operating model for the Data & Analytics capabilities.
- Design a detailed delivery and implementation roadmap.
- Define the required roles, skills and expertise (and start recruiting!)
- Establish the Data & Analytics operating model.
- Determine the required technical, software and data requirements.
- Analyse the data quality, availability and suitability.
Technology and infrastructure
Key outcome: To set up the necessary processes and structures to support the Data & Analytics function.
- Perform a technology and data capability maturity assessment.
- Conduct a technology gap analysis.
- Ensure all access to data sources are available and can be easily accessed and integrated into the data architecture.
- Ensure that the current data architecture is equipped with the necessary tools and technology to support turning the use case into tangible outcomes.
- Build the appropriate ETL pipelines and data quality checks.
Analytics and execution
Key outcome: To turn use cases into tangible outcomes and solutions.
- Identify the insights and types of analytics to use in the use cases.
- Apply analytics and machine learning techniques to build fit-for-purpose reporting solutions.
- Ensure reporting solutions and other solutions are user-friendly and represent the requirements of the stakeholders.
- Train users and continue to showcase the benefits of using analytics to the broader organisations
Written By: Christina Evans, Kim Quiñones and Thomas Maas