INDUSTRY
Postal
SERVICES
Data & Analytics
Key Takeaways
Company Overview
As Australia’s leading logistics and integrated services business, the company provides reliable and affordable postal, retail, financial and travel services across the country to more than 12.4 million delivery points.
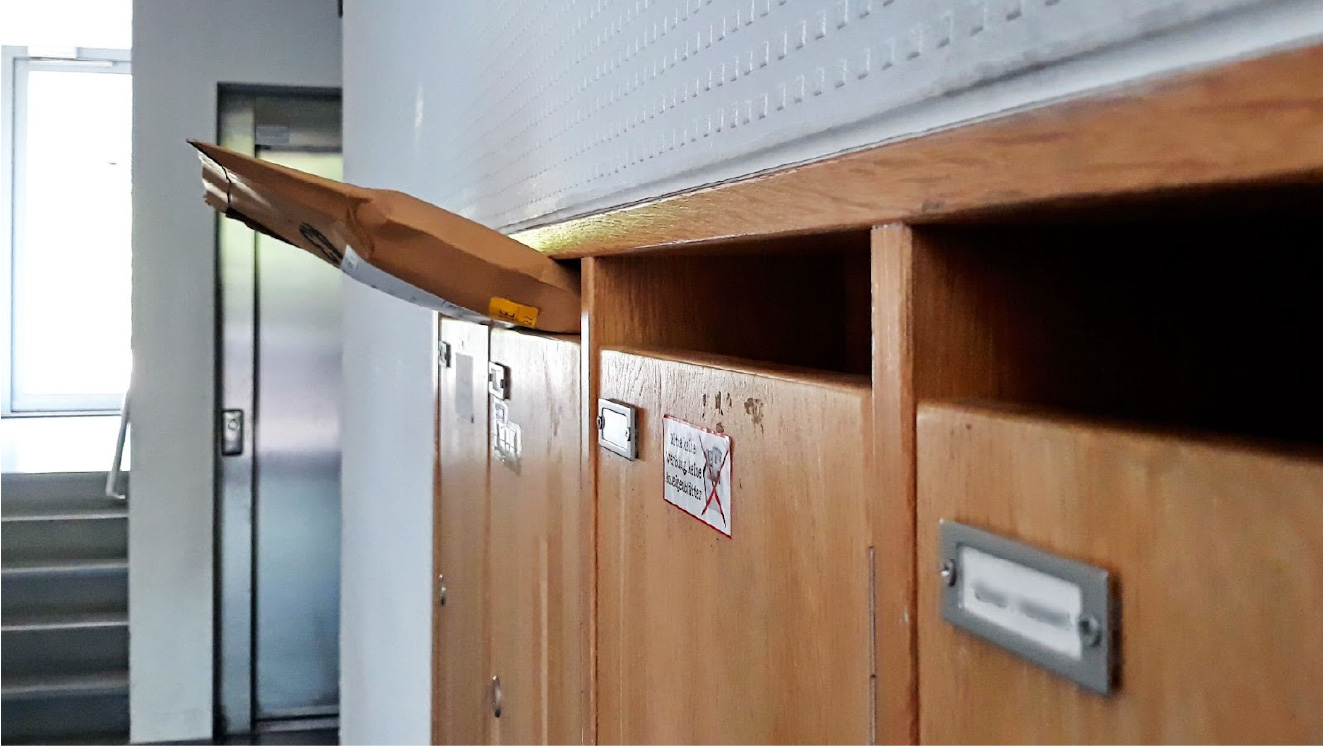
The Problem
The company had adopted the Google Cloud Platform as a cloud provider for data and insights. Over a period of time, their internal teams developed key modules and components essential to a scalable cloud Data Lake, using dbt in BigQuery for ELT pipelines. The company has also established Looker as their enterprise business intelligence platform. Their Digital and Data teams were looking to leverage their existing Google Cloud capability to uplift existing volume and revenue reporting while creating new analytics and reporting solutions in refund and pricing & yield business domains on Looker.
Generating existing volume and revenue reporting through SAP and Excel was a manual and time consuming process performed by business executives multiple times each month. Refund transaction data and customer master data were located in separate systems and not yet centralised in the enterprise Data Lake in BigQuery.
Mantel Group was engaged due to our proven expertise solving tough modern problems with speed, scale & volume with big data using innovative techniques on the Google Cloud Platform being a premium partner.
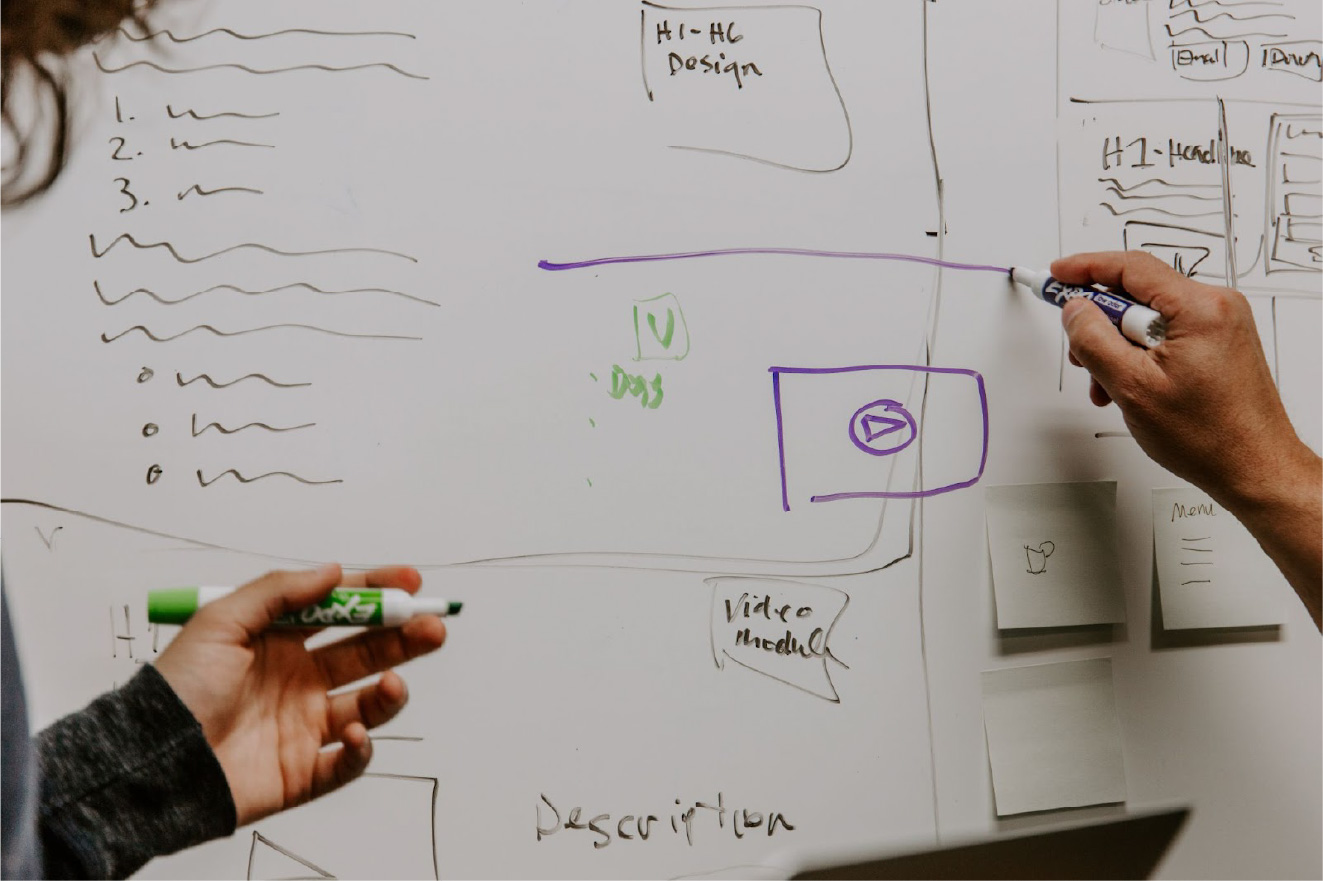
The Solution
Mantel Group onboarded transactional and customer master data into the Data Lake in BigQuery through file based ingestion patterns and third party API’s. We reverse engineered existing reporting logic in SAS and implemented new ELT pipelines in BigQuery using dbt. We also developed dashboards in Looker, modernising existing reporting solutions to be more comprehensive and performant.
Ingestion of new data sources into the Data Lake from third party APIs and external systems:
- Development of dbt models to transform transactional and customer master data, reverse engineering star schema logic from existing SAS data pipelines
- Development of Looker dashboards to uplift analytics and reporting in Refunds, Pricing & Yield, and Volume and Revenue business domains
- Assisting the company with establishing their production Looker instance and defining the process for deploying dashboards to production
Key Products/Services Used
- Google Cloud Storage
- Cloud Composer
- Cloud Datastore
- Terraform
- BigQuery
- dbt
- Looker
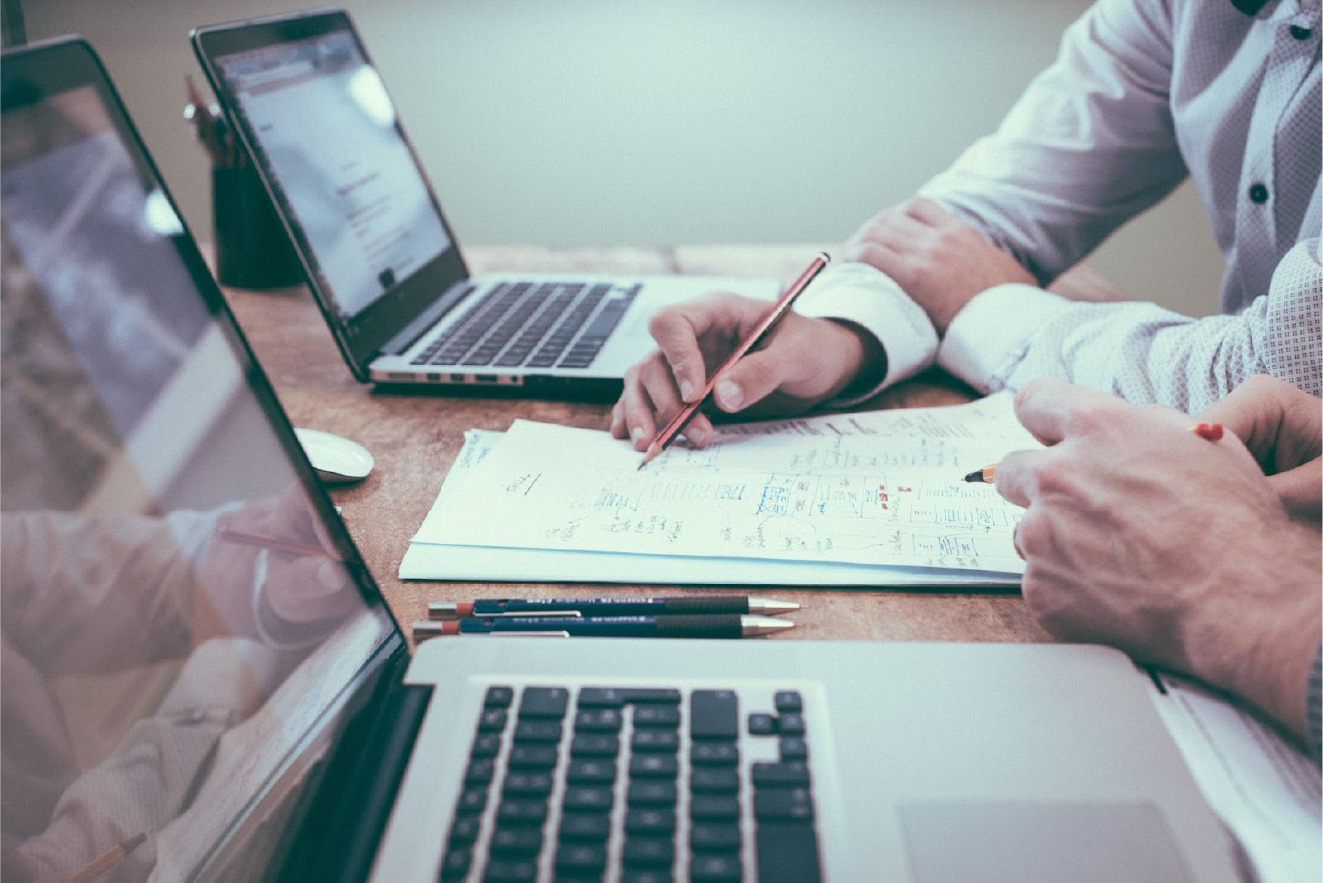
The Outcomes
Business executives can now use Looker to generate Volume and Revenue reports in seconds, rather than working through a manual and time consuming process to generate Excel reports several times a month.
Additional data sources have been centralised on the enterprise Data Lake, powering new opportunities for business intelligence and analytics on Looker.